10 Common Data Analytics Mistakes to Avoid: A Comprehensive Guide
01/06/2023 2023-01-06 21:11<strong>10 Common Data Analytics Mistakes to Avoid: A Comprehensive Guide</strong>
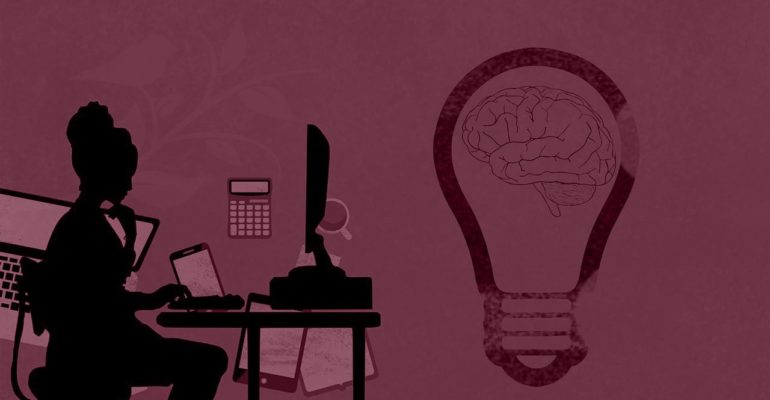
10 Common Data Analytics Mistakes to Avoid: A Comprehensive Guide
Are you looking to gain insight from data you’ve collected? Data analytics can be a powerful tool to help you make decisions and understand patterns in your data. However, if you’re not careful, it’s possible to make mistakes that can lead to wrong conclusions and bad decisions.
This comprehensive guide will walk you through 10 common data analytics mistakes to avoid, so you can make the most of the data you’ve collected. Through this guide, you’ll learn how to identify and avoid mistakes such as incorrect data sampling, incorrect assumptions, and over-reliance on data.
You’ll also be provided with best-practice methods for using data analytics to make decisions. With the help of this guide, you can ensure your data analytics journey is headed in the right direction.
Mistake 1: Incorrect Data Sampling
Data sampling is an important part of data analytics. It allows researchers to take a representative sample of a population, which can then be used for further analysis. However, if the data sampling is incorrect, it can lead to inaccurate results and unreliable conclusions. This can have a huge impact on the accuracy of data analytics.
Using incorrect data sampling can also lead to bad decision making. If the data being analyzed is not representative of the population, any decisions made based on it may be misguided or wrong.
Therefore, it is important to ensure that the data sampling is correct and representative of the population being studied. Only then can data analytics be used to draw meaningful conclusions and make reliable decisions.
Mistake 2: Incorrect Assumptions
When it comes to data analytics, incorrect assumptions can have a serious impact. Without accurate assumptions, data analysts are unable to make accurate predictions or draw valid conclusions. This can result in inaccurate reports, missed opportunities, and even costly mistakes.
For example, if a data analyst incorrectly assumes that a certain customer group is more likely to purchase a product, they may make decisions based on that assumption, only to find out it was wrong. This can lead to lost revenue and wasted resources.
On the other hand, making the right assumptions can have a big payoff. By taking the time to examine the data and make accurate assumptions, data analysts can gain invaluable insights and make informed decisions. This can help businesses make more informed choices, leading to increased profits and more accurate reports.
So, if you’re in the data analytics game, it pays to take the time to make sure your assumptions are correct. Doing so will lead to better results and more success!
Mistake 3: Over-Reliance on Data
Over-reliance on data can have a negative effect on data analytics. When data is relied upon too heavily, analytic teams may lose sight of the implications and insights the data can provide. They may become too attached to the numbers, instead of looking at the bigger picture. This can lead to data-driven decisions that lack context and fail to consider the impact on the business.
Additionally, over-reliance on data can lead to a lack of creativity and originality. Analysts may become more focused on the data than on the underlying problem or opportunity. This can lead to a lack of creativity in problem-solving, and a lack of originality in the insights the data can provide.
Lastly, the over-reliance on data can lead to a lack of communication. Analysts may become too focused on the data and lose sight of the importance of communicating their findings and recommendations to key stakeholders. This can lead to misunderstandings or a lack of buy-in from those who need to understand and act on the data.
Overall, over-reliance on data can lead to missed opportunities and missed insights. To ensure data analytics yields the most value, it is important to focus on the data, but to also remember the other key elements
Mistake 4: Not Understanding the Limitations of Predictive Modeling
Predictive modeling is a process by which predictions are made about the future based on data from the present and past. Predictive models can help us understand trends, make decisions about resource allocation, and anticipate customer needs.
However, there are some limitations to predictive modeling that must be considered. For example, predictive models can be inaccurate if the data is of poor quality or if assumptions are made that are not supported by the data. If these limitations are not taken into account, the results of data analytics can be misleading.
Additionally, predictive models can miss out on important changes in the data that can lead to inaccurate predictions. Therefore, it is important to understand the limitations of predictive models before relying on the results of data analytics. Doing so will allow organizations to make more informed decisions and help ensure that data analytics are as reliable and accurate as possible.
Mistake 5: Poorly Defined Goals
Poorly defined goals can have a major impact on data analytics results. Without a clear understanding of what you’re trying to achieve, it can be difficult to accurately evaluate how to use data to achieve those goals. Without having a clear goal in mind, it’s easy to become overwhelmed by the vast amounts of data out there and end up missing important insights.
Additionally, if the goal isn’t well defined, then the team may be unable to properly prioritize tasks and determine which data points are the most important. This can lead to data being analyzed without taking into account the overall goal, resulting in inaccurate insights and poor decision making.
Having a well-defined goal is essential in data analytics. It helps to ensure that the data is analyzed in the best way to achieve the desired outcome. By setting clear goals, teams can focus their efforts and prioritize tasks, ultimately leading to more effective data analysis and better results.
Mistake 6: Not Incorporating Human Judgment
When it comes to data analytics, it’s important to remember the importance of incorporating human judgment. Without human judgment, data analytics can become a mechanical process that overlooks important nuances and fails to consider the broader context of the data.
When human judgment is applied to data analysis, it can help to identify trends and patterns that would otherwise be missed. This can help to uncover valuable insights that can inform better decision-making and create more effective strategies.
Furthermore, the inclusion of human judgment allows data analytics to account for complex and changing dynamics that are difficult to capture with data alone. With human judgment, data analytics can be used to explore the implications of decisions, identify potential areas of risk, and anticipate the impact of future events.
All in all, the incorporation of human judgment is essential for data analytics to be successful.
Mistake 7: Not Documenting The Analysis Process:
Data analytics are becoming increasingly important in today’s world, as businesses use data to inform their decisions and drive their success. But if you’re going to make the most of this data, it’s essential to document your analysis process. Failing to do so can have serious consequences.
For one thing, it makes it much harder to replicate your findings and ensure accuracy. It also makes it difficult to return to the analysis at a later time and find out more details. Plus, if you don’t document the process, you won’t be able to share it with other stakeholders and explain how you came to certain conclusions.
So if you want to make the most out of your data analytics, make sure to document the analysis process. It may seem tedious, but it’s essential for making sure you get the best results.
Mistake 8: Ignoring Outliers:
Ignoring outliers is a common practice among data analysts. It’s a strategy used to help identify trends and patterns in data sets by eliminating the effects of extreme values. By ignoring outliers, analysts can gain a better understanding of the data they’re working with and get more accurate results.
But there are some drawbacks to this approach. For starters, ignoring outliers can sometimes lead to skewed results. If an extreme value is actually part of the trend, it won’t be taken into account and could lead to incorrect conclusions. It can also mask important insights that could be gained by leveraging the outlier.
For example, the outlier might be an indication of a problem that needs to be addressed. As such, it’s important for data analysts to be aware of the pros and cons of ignoring outliers before making a decision.
Mistake 9: Not Taking Action After Analyzing Data:
Data analytics is an incredibly powerful tool, but it can only be effective if it leads to action. If analysis is done without taking action, the data analytics process has failed. Without action, the insights gleaned from data analytics are meaningless.
Taking action after analyzing data helps businesses to identify problems, make informed decisions, and implement changes. By taking action, businesses can turn insight into tangible results. The right action taken at the right time can help businesses to stay competitive, improve operational efficiency, and increase profits.
When action isn’t taken, companies miss out on the potential benefits of data analytics. Taking action is the key to unlocking the potential of data analytics and achieving desired business outcomes.
Mistake 10: Not Understanding Data Privacy Regulations:
Companies that don’t abide by data privacy regulations can face steep fines, litigation, and reputational damage. Furthermore, companies that don’t comply with data privacy regulations run the risk of losing their customers’ trust.
As such, it is critical for companies to understand the different data privacy regulations and the implications they have for data analytics.
Companies need to be aware of the different regulations that apply in different countries and jurisdictions, and ensure that they are compliant. They should also be mindful of the different types of data they are collecting, and the security measures they are using to protect that data.
Companies that don’t understand data privacy regulations put themselves at risk of collecting and using data in a way that violates the law, leading to serious consequences.
Understanding data privacy regulations is essential for any company that wants to leverage the power of data analytics.
Conclusion
Data analytics can be an incredibly useful tool when it comes to making informed decisions and understanding patterns in your data. However, it’s important to be mindful of certain mistakes that could lead to inaccurate conclusions or bad decisions. This comprehensive guide has outlined 10 common data analytics mistakes to avoid.
By following best-practice methods and avoiding these mistakes, you can make sure that your data analytics journey is as successful as possible.
Overall, it pays to be mindful of these common mistakes in order to get the most value out of your data analytics efforts. By following the tips outlined in this guide, you can make sure that your data analytics journey is headed in the right direction.