A Guide to Avoiding Data Collection & Cleaning Errors
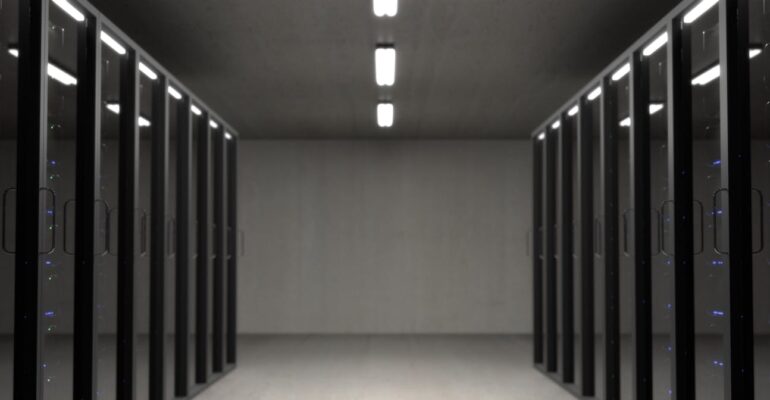
A Guide to Avoiding Data Collection & Cleaning Errors
Clean data forms the foundation for accurate analysis, reliable insights, and ultimately, sound decision-making. Unfortunately, data is rarely perfect. Errors can creep in during collection, compromising the integrity of the entire dataset. These errors, if left unchecked, can lead to misleading conclusions, wasted resources, and even negative consequences for businesses.
This guide equips you with the knowledge to avoid common data collection and cleaning errors. We’ll delve into the pitfalls to watch out for, explore effective cleaning techniques, and introduce proactive strategies for maintaining pristine data. By understanding these areas, you’ll be well on your way to harnessing the true power of your information.
Common Data Collection Errors
Data collection, the initial phase of the data lifecycle, is susceptible to several errors. Here are some of the most frequent offenders:
- Inconsistent Data Formats: Imagine a dataset where some ages are listed as numbers (e.g., 35) and others are written as text with units (e.g., “35 years old”). This inconsistency makes analysis cumbersome and error-prone. Similarly, inconsistent date formats (MM/DD/YYYY vs. DD/MM/YYYY) can create confusion.
- Missing Values: Data may be missing for various reasons, like user skips in surveys or technical glitches during collection. Missing values can skew analysis and limit the scope of insights.
- Data Entry Errors: Typos, accidental keystrokes, and human mistakes can introduce errors into data. This is especially common in manual data entry processes.
- Selection Bias: Selection bias occurs when the data collection method doesn’t represent the entire population being studied. For instance, surveying only satisfied customers online would lead to biased results, neglecting the experiences of dissatisfied customers who may not engage online.
These are just a few examples, and the specific errors encountered will vary depending on the data collection method and type of information being collected.
Essential Techniques for Avoiding Data Cleaning Errors
Once data is collected, cleaning becomes crucial. This process involves identifying and rectifying errors to ensure the data’s accuracy and usability. Here are some essential techniques:
- Data Validation Techniques: These techniques involve setting rules and limitations on data entry. For example, a form might only accept numbers for age entries or offer a dropdown menu for selecting a country. This helps prevent basic errors from the start.
- Standardizing Data Formats: Standardizing data formats ensures consistency throughout the dataset. This can involve defining specific date formats, using consistent units of measurement, and employing a predefined format for text entries.
- Utilizing Data Cleaning Tools: Thankfully, various software tools offer powerful functionalities for cleaning data. These tools can help filter out inconsistencies, sort data for easier analysis, and identify missing values. Some tools even offer data scrubbing features that automatically correct common errors based on pre-defined rules.
- Documentation: Keeping meticulous records of data collection and cleaning processes is crucial. Documentation helps identify the source of the data, the cleaning steps taken, and any transformations applied. This becomes particularly important when collaborating with others or revisiting the data for future analysis.
By combining these techniques, you can significantly reduce data cleaning errors and ensure the integrity of your information.
Proactive Strategies for Maintaining Clean Data
While reactive cleaning techniques are valuable, a proactive approach goes a long way in ensuring clean data from the outset. Here are some strategies to consider:
- Planning Data Collection Methods: Before data collection begins, clearly define what information you need and how you will collect it. This helps identify potential inconsistencies in data formats beforehand. Pilot testing your data collection methods can also reveal potential issues with missing values or selection bias.
- Implementing Data Quality Checks: Regularly review your data for errors. This can involve setting up automated data quality checks that flag inconsistencies or missing values.
- Utilizing Data Cleansing Automation: Leverage technology to streamline data cleaning processes. Many tools offer functionalities for automated data scrubbing based on pre-defined rules. However, it’s important to remember that automation shouldn’t replace human oversight entirely.
By fostering a culture of data cleanliness within your organization, you can minimize errors from the start and ensure the long-term health of your data infrastructure.
Conclusion
Clean data is the lifeblood of data-driven decision-making. By understanding common data collection and cleaning errors, implementing effective cleaning techniques, and adopting proactive strategies for data quality, you can ensure the information you work with is accurate, reliable, and ready to unlock valuable insights.
Remember, clean data isn’t just about avoiding errors; it’s about empowering you to make informed choices and achieve your goals. Furthermore, clean data fosters trust in data analysis and its results, leading to better collaboration and innovation within your organization.