Data Governance for Data-Driven Decisions: A Practical Guide for Data Analysts
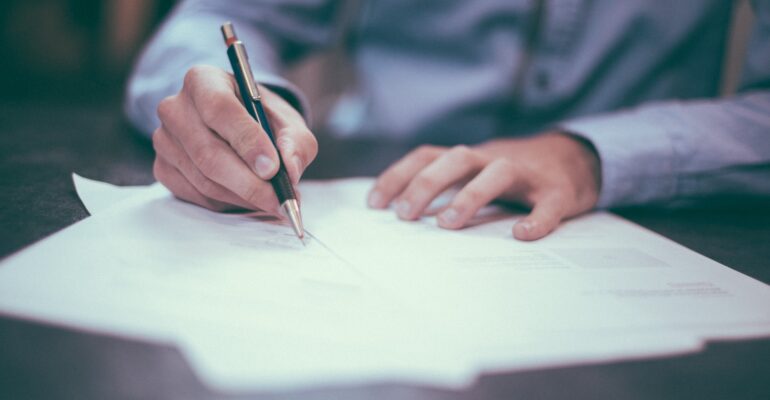
Data Governance for Data-Driven Decisions: A Practical Guide for Data Analysts
In today’s data-driven world, data analysts play a crucial role in extracting valuable insights from an ever-increasing volume of information. However, the effectiveness of these insights hinges on the quality, reliability, and accessibility of the data being analyzed.
Data governance, the process of managing and overseeing the availability, usability, integrity, and security of data, is essential for ensuring data-driven decisions.
This guide provides data analysts with a practical understanding of data governance principles and offers actionable steps to implement data governance practices in their daily work.
Key Principles of Data Governance
Data governance encompasses a set of principles that guide the management and utilization of data within an organization. These fundamental principles ensure that data is:
Data Quality: Accurate, complete, consistent, and timely data is the foundation of reliable analysis. Data analysts should advocate for data quality checks and cleansing procedures throughout the data lifecycle.
Data Security: Protecting sensitive data from unauthorized access, breaches, and misuse is paramount. Data analysts must adhere to data security policies and procedures, including encryption, access controls, and incident response plans.
Data Accessibility: Providing authorized users with timely and efficient access to data enables effective analysis. Data analysts should participate in data access reviews and ensure data is organized and documented to facilitate access.
Data Metadata: Comprehensive metadata, including data definitions, lineage, and relationships, provides context and enhances data understanding. Data analysts should contribute to metadata creation and maintenance.
Practical Steps for Data Analysts to Implement Data Governance
Data analysts can play an active role in implementing data governance practices within their organizations. Here are some practical steps:
Participate in Data Governance Initiatives: Engage with data governance committees, attend workshops, and contribute to policy development.
Advocate for Data Quality: Raise data quality concerns, participate in data cleansing efforts, and promote data quality standards.
Employ Data Documentation and Metadata Management Practices: Document data sources, definitions, and lineage, and contribute to metadata creation and maintenance.
Collaborate with Data Stewards and Stakeholders: Work with data stewards to resolve data issues, and collaborate with stakeholders to understand data needs and usage.
Utilize Data Governance Tools and Frameworks: Familiarize yourself with data governance tools and frameworks, such as data quality management tools and data governance platforms.
Examples of Data Governance in Action for Data Analysis
Data governance practices can be applied directly to enhance data analysis. Here are some examples:
Case Study 1: Implementing Data Quality Checks for Accurate Customer Analytics: A data analyst working on customer analytics implements data quality checks to identify and correct missing or inaccurate customer data, ensuring the accuracy of customer segmentation and churn analysis.
Case Study 2: Using Data Governance for Efficient Data Exploration and Cleaning: A data analyst utilizes data governance tools to discover data sources, understand data relationships, and identify data anomalies, streamlining data exploration and cleaning processes.
Case Study 3: Leveraging Data Stewardship for Collaborative Data Visualization and Reporting: A data analyst collaborates with data stewards to ensure consistent data definitions and usage across different departments, enabling accurate and reliable data visualization and reporting.
Challenges and Best Practices for Data Analysts in Data Governance
Data analysts face certain challenges in implementing data governance practices:
Data Silos and Fragmented Data Landscape: Addressing data silos and integrating data from various sources requires organizational collaboration and data governance initiatives.
Resistance to Change: Promoting a culture of data-driven decision-making and data governance may require overcoming resistance from individuals or departments accustomed to traditional data management practices.
Continuous Improvement and Adaptation: Data governance practices need to evolve as data sources, technologies, and business needs change. Data analysts should advocate for continuous improvement and adaptation of data governance policies and procedures.
Conclusion
Data governance is essential for data analysts to make informed and reliable data-driven decisions. By understanding and applying data governance principles, data analysts can play a pivotal role in ensuring data quality, security, accessibility, and compliance.
By advocating for data governance practices and collaborating with data stewards and stakeholders, data analysts can foster a culture of data-driven decision-making that drives organizational success.