Fraud Detection through Machine Learning: Safeguarding Financial Transactions
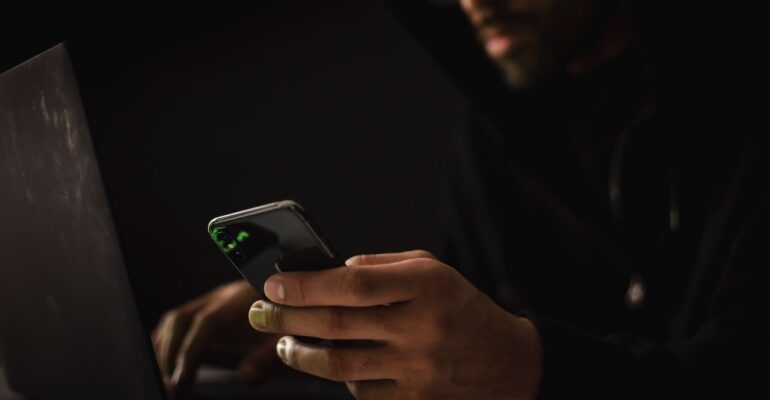
Fraud Detection through Machine Learning: Safeguarding Financial Transactions
As technology advances, fraudsters are becoming increasingly sophisticated in their methods, making it challenging for financial institutions and organizations to detect and prevent fraudulent activities.
The rise of machine learning has revolutionized fraud detection, enabling the identification of patterns and anomalies in vast amounts of data that would be nearly impossible for humans to identify.
This blog post will delve into the role of machine learning in fraud detection and explore common techniques, real-world applications, challenges, and future trends in safeguarding financial transactions.
The Role of Machine Learning in Fraud Detection
Machine learning has become a crucial tool in the fight against fraud. By analyzing vast amounts of data, machine learning algorithms can identify patterns and anomalies that may indicate fraudulent activity. These algorithms can learn from historical data, enabling them to improve their detection capabilities over time.
The importance of machine learning in fraud detection cannot be overstated. According to a report by the Association of Certified Fraud Examiners, organizations that use machine learning and artificial intelligence in their fraud detection efforts experience a 52% higher return on investment compared to those that do not.
Moreover, machine learning algorithms can process massive data sets in real-time, allowing for faster and more accurate fraud detection. This is particularly essential in the financial industry, where timely detection can mean the difference between stopping a fraudulent transaction and losing millions of dollars.
Common Machine Learning Techniques for Fraud Detection
Several machine learning techniques are commonly used in fraud detection, with random forest and logistic regression being popular choices. Random forest is an ensemble learning method that combines multiple decision trees to identify patterns in data.
This technique is particularly effective in detecting credit card fraud, as it can analyze numerous transaction attributes, such as amount, location, and time of day, to identify suspicious activity.
Logistic regression, on the other hand, is a supervised learning method that predicts the likelihood of an event occurring based on input variables. In fraud detection, logistic regression can analyze various data points, such as transaction amount, user behavior, and location, to determine the probability of fraudulent activity.
Real-World Applications of Machine Learning in Fraud Prevention
Numerous financial institutions and organizations have successfully implemented machine learning in their fraud detection efforts. PayPal, for instance, uses machine learning algorithms to analyze over 300 attributes in real-time to detect fraudulent transactions.
Another example is the Bank of America, which uses machine learning to monitor credit card transactions. Their system analyzes numerous data points, including location, amount, and time of day, to identify potential fraud. In 2020, the bank reported a 50% reduction in fraud thanks to their machine learning-powered fraud detection system.
Challenges and Considerations in Fraud Detection
While machine learning has revolutionized fraud detection, several challenges remain. One of the most significant challenges is false positives. Inaccurate fraud detection can lead to frustrated customers and unnecessary costs for financial institutions.
Another challenge is imbalanced datasets, where legitimate transactions outnumber fraudulent ones. This imbalance can make it difficult for machine learning algorithms to accurately detect fraud.
Fraudsters are also becoming increasingly sophisticated, using techniques such as “low and slow” attacks, where they make small, repeated transactions to avoid detection. Additionally, fraudsters often use stolen personal information to make transactions that appear legitimate.
Given these challenges, it’s crucial to continuously monitor and adapt fraud detection models to changing fraud patterns. Machine learning algorithms must evolve to stay ahead of fraudsters and address emerging threats.
Future Trends in Fraud Detection
As fraudsters continue to evolve their tactics, the financial industry must also innovate to stay ahead. Emerging technologies and trends are set to revolutionize fraud detection, making it faster, more accurate, and more efficient. Here are some of the most promising developments on the horizon:
Artificial Intelligence (AI) and Machine Learning (ML)
AI and ML are already being used in fraud detection, but their potential is far from fully tapped. As these technologies continue to advance, we can expect to see even more sophisticated fraud detection systems that can learn from vast amounts of data, identify patterns, and make predictions.
One area where AI and ML are likely to have a significant impact is in the detection of micro-transactions. These are small, seemingly innocuous transactions that can be used to test the validity of a payment card or to launder money.
AI-powered systems can analyze transaction data in real-time to identify patterns that may indicate micro-transactions, allowing financial institutions to flag these transactions and prevent fraud.
Conclusion
The fight against fraud is an ongoing one, and financial institutions must continue to innovate to stay ahead of fraudsters. Emerging technologies and trends like AI, real-time fraud detection, collaborative fraud detection, biometric authentication, and advanced data analytics are set to revolutionize fraud detection, making it faster, more accurate, and more efficient. By embracing these developments, financial institutions can protect their customers and prevent fraud in the digital age.